From the author: Context, Hedging, and Caveats
I wanted to write a slick illustrated explainer. This is not that. It still includes a high level explanation of the FEP but its focus is my journey of learning about it to my satisfaction.
The target audience for this one is "me before I started", or anyone curious about my process.
Thanks to Tasshin, Daniel Friedman, Ran Wei for discussion, revision and helpful comments.
Introduction
I like analogies between entities at different scales. Cells, organs, sub-personalities, individual people, families and teams, congregations and larger companies, cities, countries and networks etc. It's not that they're all strongly analogous, but there are principles that apply across scales, like many properties of networks, or game theory, or natural selection.
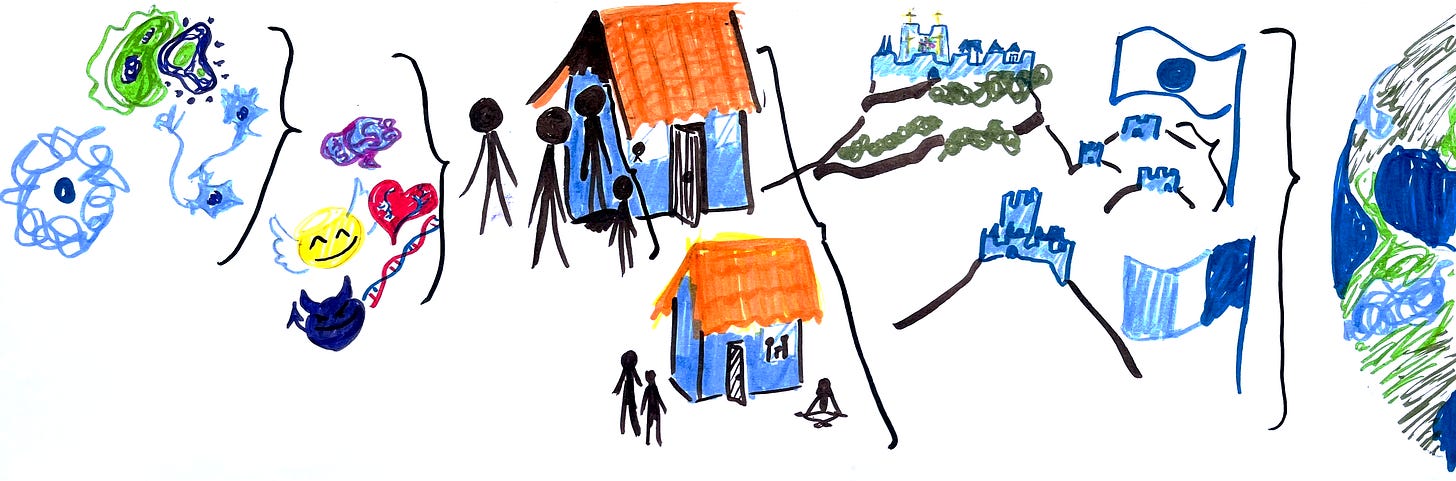
These analogies seemed to suggest an underlying language to talk about entities and their interactions across scales, but I couldn't quite articulate it myself. Over time, I kept spotting something called "the free energy principle" applied to very diverse settings. "This could be it!"
The FEP is famously hard to get into, but after a few waves of running into it, reading a few explainers, and forgetting about it - I think it stuck well enough that I can talk about it.
Super broad strokes
The FEP basically says that the fundamental drive of self-organizing systems is to minimize surprise.
By self-organizing systems, let us picture things that don’t dissipate into the background. A drop of ink in a water cup dissipates - a cell doesn’t, a tree doesn’t, an anthill doesn’t, etc.
Surprise is destabilizing. And in this framing, destabilization is surprise. Destabilizing events knock you out of homeostasis (kill you). So you either want to get good at anticipating danger in complex surroundings, or to change your environment to one that supports your homeostasis.
“What about seeking surprising information or novelty?” We minimize surprise over time, so we’re trading off exploration and exploitation. Anticipating danger requires mapping the unknown to better predict the world in the future. Same for changing your environment, you want to be able to tell what the consequences of your actions will be.
“If we’re minimizing surprise, why don’t we lock ourselves in a dark room?” A dark room just isn’t an environment that supports your homeostasis very much. It’s not somewhere you’re adapted to. So you would feel compelled to turn the light on, to gather information and change your environment for the better..
By an environment that supports homeostasis, I mean one that satisfies the conditions needed for survival like the right temperature range, available food and drink, and no physical danger. We can stretch this concept of homeostasis to include our preferences and tastes, so in a minimally surprising environment I’d be surrounded by loved ones, and by art and stimuli perfectly suited to my current mood.
Associated to the FEP are other names like "active inference" and "Bayesian brain" and "predictive processing" which I think this diagram from Opentheory’s "Seed Ontologies" does a good job of distinguishing..
For reference later, active inference assumes these “ideal conditions for homeostasis” are encoded in the world models of organisms, and calls them “preferences”. I think they might explain too much, but more on that later.
Despite not being fully satisfied with the role of preferences, I thought there were enough new insights for me that the fact that things minimize surprise became a lens in my repertoire.
I find it relieving to know there is plausible formal ground for the analogies I was already using to make sense of things as embedded in (instead of separate from) their environment. Analogies based on information and computation and networks and so on. Plus, it accords with the wispy graph-like visualizations of the history of human systems that my mind tends to like.
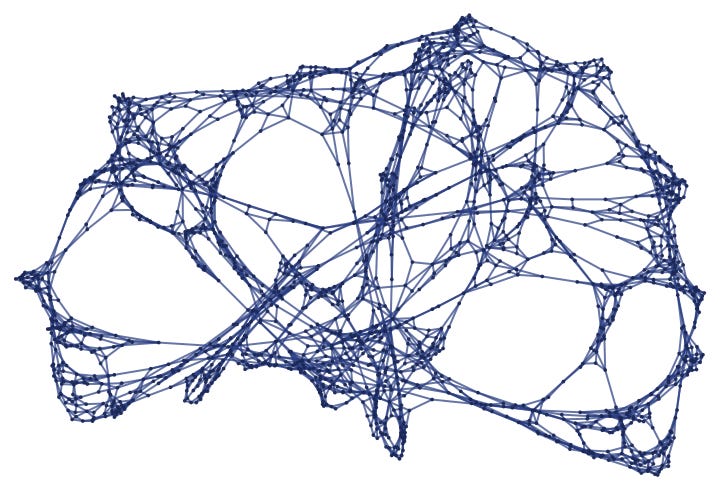
The "FEP" posts
Why I started writing
In January 2024 I met my friend Tasshin in Berkeley for lunch and a hike and basically gave him my download of the FEP, as well as how I understand a bunch of concepts from Buddhism and philosophy and woo more or less in terms of it. He quickly adopted it and invited me to collaborate on a blogpost giving a high-level explanation and then exploring some examples. I said "yes but I haven't gone into all the math".
Impostor syndrome sets in...
"Who am I to write a post explaining active inference?" I was confused about its philosophical status as a principle, and whether it was even useful without the math.
TLDR: I think it works well even just as a language to make analogies with, or a set of constraints and assumptions to tell stories (make hypotheses) about how unknown systems may work.
Philosophical status of the FEP
This "summary for a wide audience" helped me by clarifying that the FEP is not a theory, it's a principle based on which we can formulate Bayesian mechanical theories that partition a system into "things'' that are separate from but coupled to others by beliefs. Just like the Hamiltonian principle of least action gives rise to classical mechanics and lets us predict the motion of rigid particles, this lets us predict the behavior of things that do Bayesian inference.
I gave writing the post a try anyway. Requested feedback from friends, and realized it was underspecified and hand-wavy in many ways, and that it didn't really work as an explanation for someone new to the FEP.
Approaches to explaining the FEP
I really wanted to be able to explain this well so I dove into Parr's Active Inference book. The book gives two paths into active inference: the "low road" which takes the Bayesian brain hypothesis as a given and expands Bayesian inference to include actions, and the "high road" which starts only with the observation that things that don’t dissipate must have boundaries.
The "low road" in the book explained the math first by motivating it with the Bayesian brain hypothesis. I thought I might get the math out of the way first so I wrote 80% of an illustrated "low road to active inference" for dummies. I showed it to my girlfriend. She felt like "it answered a question she didn't have", and I realized I wasn't clear on why my target audience was.
Jake Orthwein, who has also assigned himself the task of concisely explaining active inference, told me some people are motivated and get it, and some don't really feel like they were looking for an answer to begin with. Tasshin likes to write to his younger self, it takes the pressure off. I don't know why I didn't feel like this wasn't good enough.
I also spent a while surveying other ways people explained active inference and the FEP. I liked this lecture by Maxwell Ramstead. He motivates it as a way to make sense of nested multi-scale systems. He starts by saying that things must resist dissipation, then he explains predictive processing because the brain is a good central example, then Bayesian inference, and finally defines Markov blankets and variational free energy.
I have also since found these two illustrated posts from 2018 which go into the math of the FEP a bit and its implementation as active inference quite a bit more.
Narrowing down my scope, I wrote 80% of a "short" explainer for "technical people" explicitly addressing common confusions. I didn’t finish because I realized that for a post like that, I’d really like to compare active inference with reinforcement learning, but didn’t have the steam to fully go through with it.
I did find a couple of interesting posts from a perspective of model-based RL where epistemic and pragmatic value are usually two separate learning objectives, while in active inference they’re unified. In this sense, it seems that RL may be strictly more general than active inference for solving problems of reward-maximization because it splits pragmatic and epistemic utility. I wonder if the value of active inference in comparison lies in making stronger assumptions that apply to real self-organizing systems in the world.
Limitations of active inference
In active inference, the description of an organism’s “homeostatic goal”, or its “preferences” would be encoded in the priors of its generative model. I think my biggest gripe with active inference right now is how much work the preferences seem to do, and how little is said about them. The framework is elegant - “things just minimize free energy” - because the complexity is kicked down to the preferences.
“Why does Garry dress up as Sonic the hedgehog every Tuesday morning? Why was this idiosyncratic action taken?”, “Oh that’s still perfectly explained, it’s just part of Garry’s preferences. He would find not being Sonic on Tuesdays super surprising.” Where do preferences come from? How are they learned? What else can we say about them?
In the case of humans, preferences like existential conditions (temperature range, food, drink, social embededness) seem pretty stable. Then we have really low level inductive biases that shape the development of cognition - core knowledge in 4 systems that are present from early on which represent objects, actions, number, and space (as well as maybe a fifth for social partners). Beyond that, (and I didn’t exhaustively investigate) most preferences seem incredibly dependent on developmental and cultural context. There seems to be interest in modeling the emergence of these across time scales.
It seems to me that preferences might not be that problematic when they are easily describable as in the case of existential conditions for most living things. I think they’re most problematic when the organism’s idiosyncratic preferences have a lot of bearing on the world, as in the case of humans, groups of humans, and potentially LLMs.
Conclusion
I think to do an amazing job at communicating the value I see in the FEP and active inference as frames, I'd want a curated list of examples. As well as a few “case studies” of looking at unknown systems through the FEP to make sense of them. I’m open to realizing I actually don’t get that much more value from the lens than I get from applying, say, reinforcement learning to the same setting.
I think I could start gathering examples by just reading a bunch of applications of active inference to different fields like morphogenesis and social systems and art, but that sounds like a lot of work. A slightly lower activation energy version of that would be to listen to presentations on Active Inference Institute's youtube channel, but still a considerable time investment. There’s also this live list of code implementations.
Despite my resistance to gather examples, I’m glad that I got to dive deep on some pretty fundamental conceptual apparatus and a bit of the philosophy of science around it. I could go further and read about the ways active inference was updated over time to respond to criticism, but that's not burning a very big hole in my curiosity right now.
I hope this was useful. If we're alike, there's a chance you got some understanding of the FEP from my post directly. I also hope this was interesting from an autodidactics perspective, as well as for the tiny niche of FEP-communicators.
Even if it’s not the whole story, I think the FEP adds magic to my daily life. It tucks away the complexity of preferences inside each organism without much explanation, yes. But by doing that, it lets us conceive of things in huge nested multi-scale systems as not fundamentally separate, and optimizing a single normative quantity in their perception, learning, action, and planning.
And that’s a cool way of looking at things that can pay off sometimes maybe!
Sources
Intuitions on predictive coding and the free energy principle
Making Sense of Active Inference: Optimal Control Without Cost Function
Another attempt to rationalize Expected Free Energy: Insights from Reinforcement Learning
Bonus Glossary
Free Energy Principle (FEP): A theoretical framework that describes how living systems maintain order and resist entropy by minimizing free energy, which is related to surprise or prediction error.
Active Inference: A process by which organisms minimize free energy by continuously updating their models of the world through perception and action.
Bayesian Brain: The hypothesis that the brain functions as a Bayesian inference machine, constantly updating its beliefs based on sensory input.
Predictive Processing: A theory suggesting that the brain continuously generates and updates a model of the environment to predict sensory input and minimize prediction errors.
Homeostasis: The process by which a system maintains internal stability despite external changes.
Markov Blanket: A concept from statistics defining the boundary that separates the internal states of a system from external states, allowing interaction without dissolution.
Surprise (in FEP context): An information-theoretic quantity representing the difference between expected and actual sensory input; minimizing surprise helps maintain stability.
Bayesian Inference: A method of statistical inference in which Bayes’ theorem is used to update the probability of a hypothesis as more evidence or information becomes available.
Variational Free Energy: An approximation of free energy used in Bayesian inference to make calculations tractable; it balances model complexity with accuracy.
Expected Free Energy: The predicted variational free energy over time, guiding decisions that balance exploration and exploitation to minimize future surprise.
Generative Model: A model used to generate predictions about sensory inputs based on internal states and beliefs.
Hamiltonian Principle of Least Action: A principle in physics stating that the path taken by a system between two states is the one for which the action is minimized.
Homeostasis: The process by which a system maintains stability while adjusting to conditions that are optimal for survival.
Entropy: A measure of disorder or randomness in a system, often related to the second law of thermodynamics.
Cybernetics: The study of control and communication in animals and machines, focusing on feedback loops and regulatory systems.
Epistemic Value: The value derived from reducing uncertainty or gaining knowledge about the world.
Pragmatic Value: The value derived from achieving goals or fulfilling practical needs.
Reinforcement Learning: A type of machine learning where an agent learns to make decisions by receiving rewards or punishments for its actions, aiming to maximize cumulative reward.
Nice!! I've generally found myself a bit skeptical of the "free energy minimization" framework of cognition, as it has looked to me like its spread is powered as much by memetics as it is by true explanatory power. I also failed to understand the basic idea of why it would ever be a good strategy to tailor my actions so as it align reality with my predictions of it.
I found this article which you linked to be very helpful in starting to resolve some of this: https://latentobservations.substack.com/p/making-sense-of-active-inference
The high level idea almost looks to me like its saying that by doing planning in "observation space" instead of "model space," I can achieve some robustness to model misspecification. Which makes some intuitive sense!
What I haven't understood yet is how the framework addresses learning (which probably means I haven't understood all that much, haha). Maybe this is similar to your complaint about the prior being overly determinative... Definitely interested to spend some more time digging into a couple of these papers!